■ Feature
► Description:
Silimanite brick is a kind of refractoriness which made by silimaniteand other minerals. And silimanite can be transformed to be mullite above 1500℃ high temprature.
► The main applications are:
1,Rider arches;
2,Forehearth superstracture;
3,Bushing for rochwool and fiber glass;
4,High temprature furnaces, such as glass melting furnace,etc .
► Advantages:
1,Corrosion-resistant;
2,Dense structure;
3,Good thermal shock resistance.
Silimanite brick is a kind of refractoriness which made by silimaniteand other minerals. And silimanite can be transformed to be mullite above 1500℃ high temprature.
► The main applications are:
1,Rider arches;
2,Forehearth superstracture;
3,Bushing for rochwool and fiber glass;
4,High temprature furnaces, such as glass melting furnace,etc .
► Advantages:
1,Corrosion-resistant;
2,Dense structure;
3,Good thermal shock resistance.
■ Technical Data
Item | GXS-50 | GXS-60 | GXS-62 | |
Chemical composition | Al2O3 | ≥50 | ≥60 | ≥62 |
SiO2 | ≤45 | ≤38 | ≤33 | |
Fe2O3 | ≤1.2 | ≤1.0 | ≤1.0 | |
Apparent Porosity% | ≤18 | ≤18 | ≤17 | |
Bulk Density g/cm3 | ≥2.4 | ≥2.5 | ≥2.55 | |
Cold Crushing Strength Mpa | ≥60 | ≥60 | ≥60 | |
0.2Mpa Refractoriness Under Load T0.6 ℃ | ≥1550 | ≥1600 | ≥1650 | |
Permanent Linear Change On Reheating (%)1500℃X2h | ±0.1 | ±0.1 | ±0.1 | |
Thermal Shock Resistances 100℃ water cycles | ≥20 | ≥20 | ≥20 | |
Reversible Thermal Expansion 1000℃ | 0.55 | 0.6 | 0.6 | |
Pyrometric Cone Equivalent S.C. SK | 35 | 36 | 38 | |
Range of Application | Rider Arches | Superstructure forehearth | Superstructure forehearth |
■ About Us
development history »
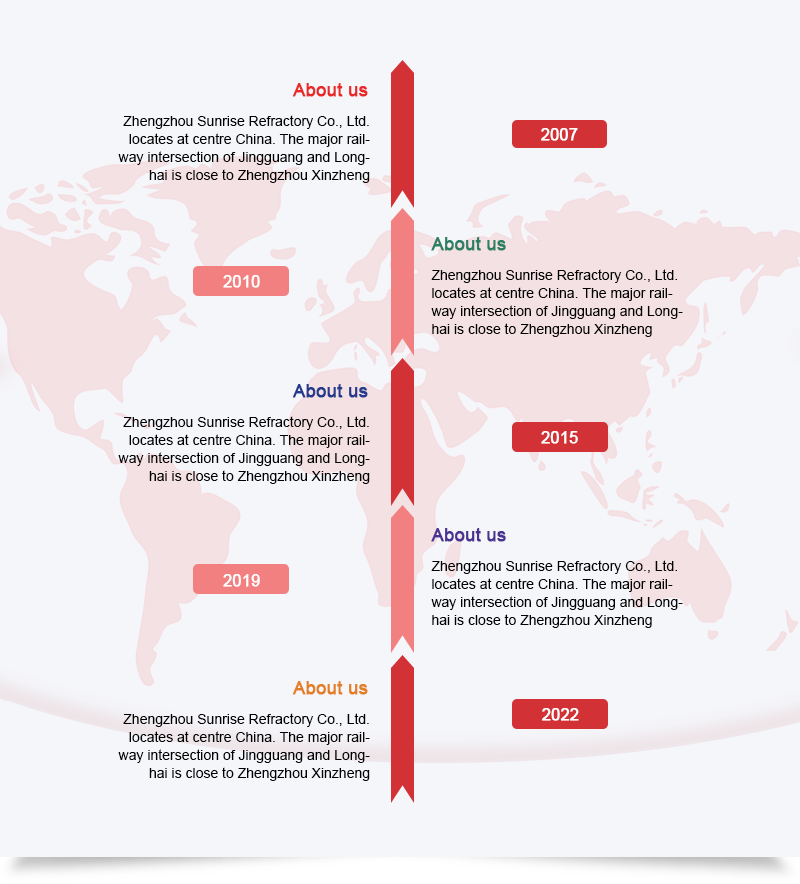
Factory strength »
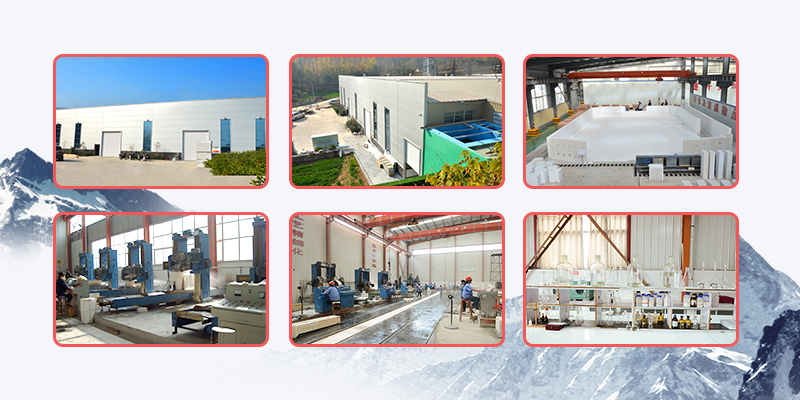
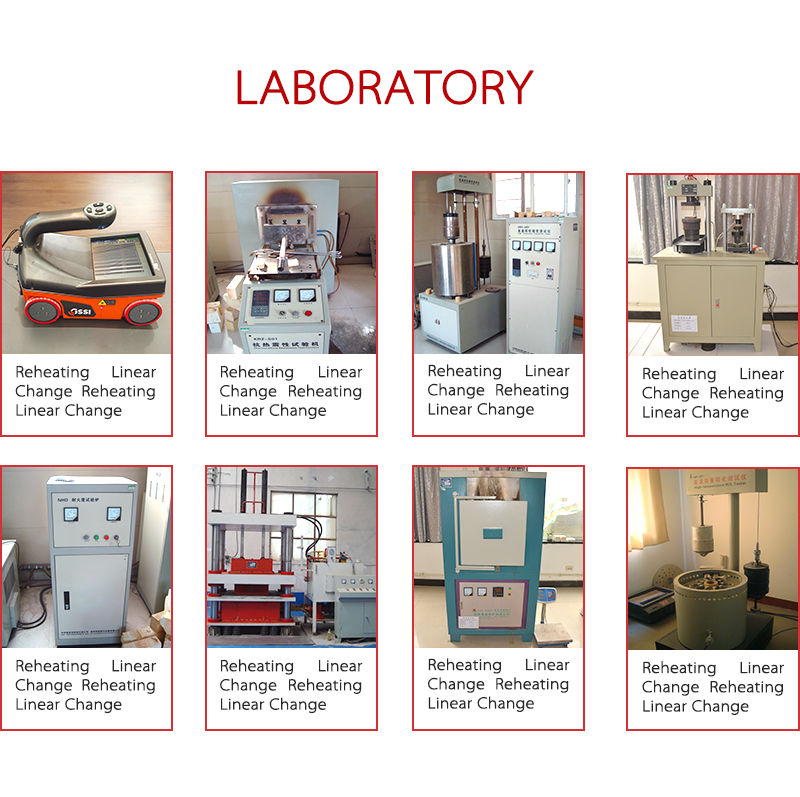
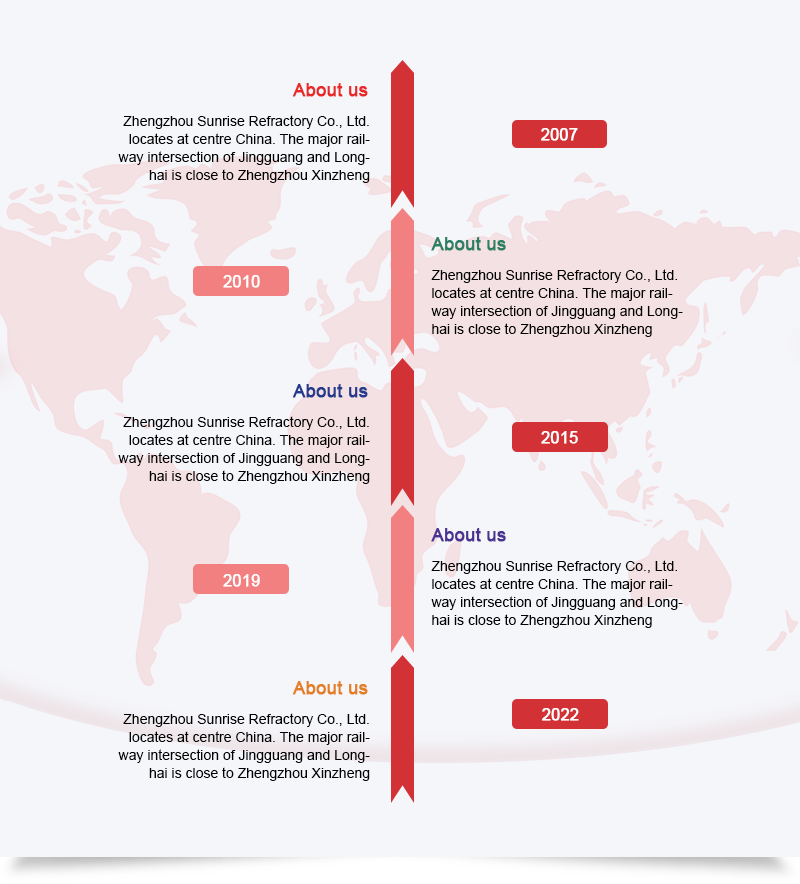
Factory strength »
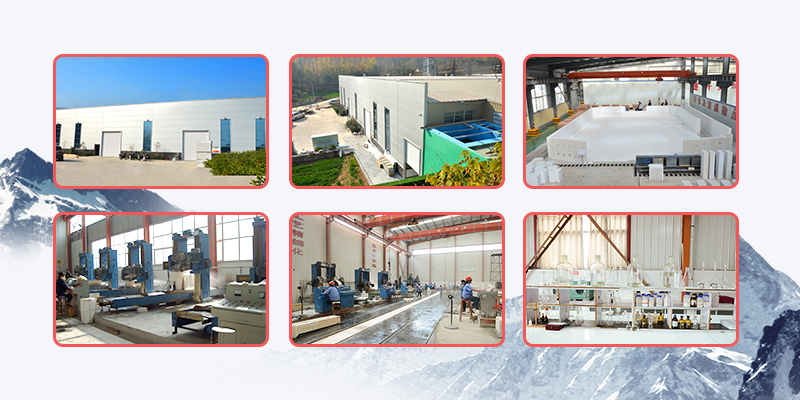
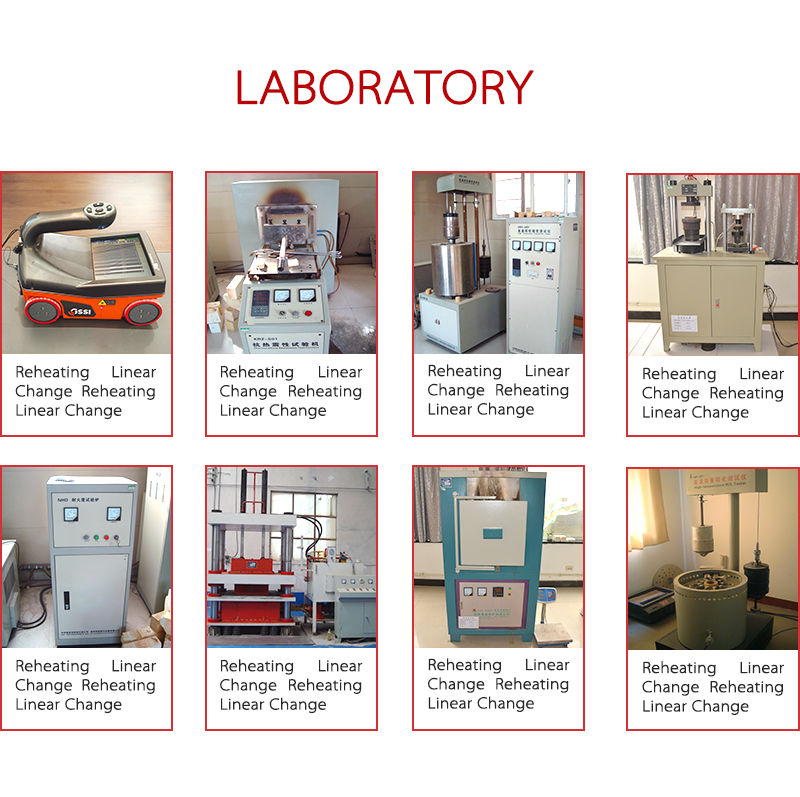